Team
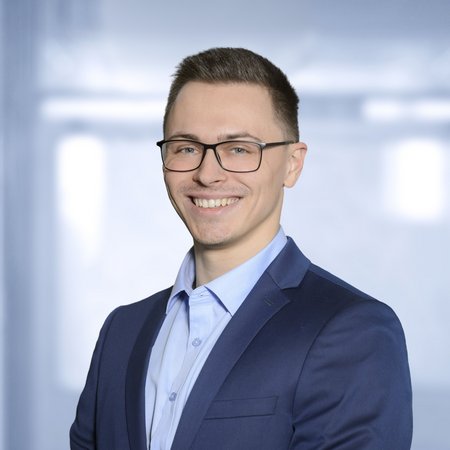
Wissenschaftlicher Mitarbeiter
Jan Laufer, M. Sc.
- Raum:
- R09 R02 H42
- Telefon:
- +49 201 18-34087
- E-Mail:
- Jan.Laufer (at) paluno.uni-due.de
- X.509 Cert:
- Nutzerzertifikat auf DFN.de
- Sprechstunde:
- Flexibel - Anmeldung per Email
- Social Media:
- Adresse:
- Universität Duisburg-Essen, Campus Essen
Fakultät für Informatik
Lehrstuhl für Wirtschaftsinformatik und Softwaretechnik
Universitätsstr. 9
45141 Essen - Autorenprofile:
- ORCID
- Google Scholar
Lebenslauf:
Berufserfahrung
- Seit Oktober 2024: Wissenschaftlicher Mitarbeiter (Vollzeit) am Lehrstuhl für Wirtschaftsinformatik und Softwaretechnik (Lehrstuhlinhaber: Prof. Dr. Stefan Eicker) an der Universität Duisburg-Essen
- Oktober 2023 - September 2024: Wissenschaftlicher Mitarbeiter (Vollzeit) am Lehrstuhl Software Systems Engineering (Lehrstuhlinhaber: Prof. Dr. Klaus Pohl) an der Universität Duisburg-Essen Duisburg-Essen
- Januar 2021 - September 2023: Wissenschaftlicher Mitarbeiter (Teilzeit) am Lehrstuhl Software Systems Engineering (Lehrstuhlinhaber: Prof. Dr. Klaus Pohl) an der Universität Duisburg-Essen Duisburg-Essen
- Mai 2018 - Dezember 2020: Studentische Hilfskraft am Lehrstuhl Software Systems Engineering (Lehrstuhlinhaber: Prof. Dr. Klaus Pohl) an der Universität Duisburg-Essen
Studium
- Oktober 2020 - Juli 2024: Studium der Wirtschaftsinformatik (M. Sc.) an der Universität Duisburg-Essen
- Masterarbeit: "An empirical user study of an approach for explaining online reinforcement learning decisions of adaptive systems"
- Oktober 2017 - Oktober 2020: Studium der Wirtschaftsinformatik (B. Sc.) an der Universität Duisburg-Essen
- Bachelorarbeit: "Modellierung von Datenschutzgefährdungen im Fog-Computing anhand von Fallbeispielen aus dem EU-Forschungsprojekt FogProtect mithilfe der Modellierungssprachen UMLsec und SysML-Sec"
Ehrungen und Auszeichnungen:
- Stipendiat Deutschlandstipendium (UDE-Stipendium) im Sommersemester 2023
- Stipendiat Deutschlandstipendium (UDE-Stipendium) im Wintersemester 2020/2021 - Sommersemester 2021 (Förderer: Dr. Heinz-Horst Deichmann Stiftung)
Forschungsgebiete:
Aktuelle Forschungsgebiete:
Meine Forschungsinteressen liegen im Bereich der Generative AI (GenAI), mit einem besonderen Fokus auf deren Nutzung und Interaktion in Realwelt-Anwendungen. Beispiele hierfür sind Prompting, Voice, Image und weitere Modalitäten. Besonders spannend ist die aufkommende physische Interaktion von GenAI mit sich selbst, Menschen und der Umwelt.
Dementsprechend ist ein zentrales Thema meiner Interessen das GenAI Embodiment. GenAI verleiht dabei einer physischen Form, wie etwa einem Roboter, bisher nie dagewesene Intelligenz. Die Zusammenarbeit zwischen Mensch und Embodied GenAI eröffnet hierbei ein faszinierendes Forschungsfeld. Dabei gilt auch die das Feld der AI Agents zu beachten, die zunehmend komplexere Aufgaben in verschiedenen Domänen übernehmen können.
Abgerundet wird meine Forschung durch den Fokus auf Explainable AI, da Transparenz und Nachvollziehbarkeit entscheidende Faktoren für Akzeptanz, Vertrauen und eine effektive Zusammenarbeit zwischen Mensch und KI darstellen.
Frühere Forschungsgebiete:
- (Self-)Adaptive Systems
- Data Protection
Publikationen:
- Metzger, Andreas; Laufer, Jan; Feit, Felix; Pohl, Klaus: A User Study on Explainable Online Reinforcement Learning for Adaptive Systems. In: ACM Trans. Auton. Adapt. Syst. (TAAS), Jg. 19 (2024) Nr. 3. doi:10.1145/3666005BIB DownloadKurzfassungDetails
Online reinforcement learning (RL) is increasingly used for realizing adaptive systems in the presence of design time uncertainty because Online RL can leverage data only available at run time. With Deep RL gaining interest, the learned knowledge is no longer represented explicitly but hidden in the parameterization of the underlying artificial neural network. For a human, it thus becomes practically impossible to understand the decision-making of Deep RL, which makes it difficult for (1) software engineers to perform debugging, (2) system providers to comply with relevant legal frameworks, and (3) system users to build trust. The explainable RL technique XRL-DINE, introduced in earlier work, provides insights into why certain decisions were made at important time steps. Here, we perform an empirical user study concerning XRL-DINE involving 73 software engineers split into treatment and control groups. The treatment group is given access to XRL-DINE, while the control group is not. We analyze (1) the participants’ performance in answering concrete questions related to the decision-making of Deep RL, (2) the participants’ self-assessed confidence in giving the right answers, (3) the perceived usefulness and ease of use of XRL-DINE, and (4) the concrete usage of the XRL-DINE dashboard.
- Metzger, Andreas; Bartel, Jone; Laufer, Jan: An AI Chatbot for Explaining Deep Reinforcement Learning Decisions of Service-Oriented Systems. In: Monti, F.; Rinderle-Ma, S.; Ruiz Cortés, A.; Zheng, Z.; Mecella, M. (Hrsg.): International Conference on Service-Oriented Computing (ICSOC). Springer, 2023, S. 323-338. doi:10.1007/978-3-031-48421-6_22BIB DownloadDetails
- Smolka, Sven; Laufer, Jan; Mann, Zoltán Ádám; Pohl, Klaus: UMLsec4Edge: Extending UMLsec to model data-protection-compliant edge computing systems. In: 2022 48th Euromicro Conference on Software Engineering and Advanced Applications (SEAA). Gran Canaria, Spain 2022, S. 418-425. doi:10.1109/SEAA56994.2022.00072BIB DownloadDetails
- Zmiewski, Sascha Sven; Laufer, Jan; Mann, Zoltán Ádám: Automatic online quantification and prioritization of data protection risks. In: Proceedings of the 17th International Conference on Availability, Reliability and Security (ARES). Association for Computing Machinery (ACM), Vienna, Austria 2022. doi:10.1145/3538969.3539005BIB DownloadKurzfassungDetails
Data processing systems operate in increasingly dynamic environments, such as in cloud or edge computing. In such environments, changes at run time can result in the dynamic appearance of data protection vulnerabilities, i.e., configurations in which an attacker could gain unauthorized access to confidential data. An autonomous system can mitigate such vulnerabilities by means of automated self-adaptations. If there are several data protection vulnerabilities at the same time, the system has to decide which ones to address first. In other areas of cybersecurity, risk-based approaches have proven useful for prioritizing where to focus efforts for increasing security. Traditionally, risk assessment is a manual and time-consuming process. On the other hand, addressing run-time risks requires timely decision-making, which in turn necessitates automated risk assessment. In this paper, we propose a mathematical model for quantifying data protection risks at run time. This model accounts for the specific properties of data protection risks, such as the time it takes to exploit a data protection vulnerability and the damage caused by such exploitation. Using this risk quantification, our approach can make, in an automated process, sound decisions on prioritizing data protection vulnerabilities dynamically. Experimental results show that our risk prioritization method leads to a reduction of up to 15.8% in the damage caused by data protection vulnerabilities.
- Lachner, Clemens; Laufer, Jan; Dustdar, Schahram; Pohl, Klaus: A Data Protection Focused Adaptation Engine for Distributed Video Analytics Pipelines. In: IEEE Access, Jg. 10 (2022), S. 68669-68685. doi:10.1109/ACCESS.2022.3185990BIB DownloadDetails
- Laufer, Jan; Mann, Zoltán Ádám; Metzger, Andreas: Modelling Data Protection in Fog Computing Systems using UMLsec and SysML-Sec. In: ACM IEEE International Conference on Model Driven Engineering Languages and Systems Companion (MODELS-C). Fukuoka, Japan 2021, S. 777-786. doi:10.1109/models-c53483.2021.00124BIB DownloadDetails
- Mann, Zoltán Ádám; Kunz, Florian; Laufer, Jan; Bellendorf, Julian; Metzger, Andreas; Pohl, Klaus: RADAR: Data Protection in Cloud-Based Computer Systems at Run Time. In: IEEE Access, Jg. 9 (2021), S. 70816-70842. doi:10.1109/ACCESS.2021.3078059BIB DownloadDetails
Vorträge:
- Laufer, Jan: Automatic online quantification and prioritization of data protection risks, FoMSESS-Jahrestreffen, 05.10.2022, Virtual. Details
- Laufer, Jan: Automatic online quantification and prioritization of data protection risks, 17th International Conference on Availability, Reliability and Security, 24.08.2022, Vienna, Austria. Details
- Laufer, Jan: Modelling Data Protection in Fog Computing Systems using UMLsec and SysML-Sec, 13th System Analysis and Modelling Conference, 11.10.2021, Virtual. Details
Lehrveranstaltungen:
Betreuung von
- Seminararbeiten:
- Gemeinsame Betreuung von Themen in den Bereichen “Generative AI im Alltag", “Generative AI für Künstler*innen und Kreative” sowie “AI Kollaboration” (WiSe 2024/2025)
- "Gegenüberstellung unterschiedlicher Ansätze zur Modellierung von Security Patterns" (SoSe 2021)
- "Attack Trees: Möglichkeiten und Einsatzgebiete" (SoSe 2022)
- "Übersicht über DevSecOps Methoden und Metriken" (WiSe 2022/2023)
- "Resolving Causal Confusion in Deep Reinforcement Learning: An Introduction to Current Approaches" (WiSe 2023/2024)
- "Identifizierung von ChatbotEvaluationsmöglichkeiten" (SoSe 2024)
- "Identifizierung von Einsatzmöglichkeiten von Large Language Models im Requirements Engineering" (SoSe 2024)
- Bachelorprojekten:
- Gemeinsame Betreuung von Themen in den Bereichen “Generative AI als Lernunterstützung”, “Generative AI zur menschlichen Verhaltenssimulation”, “Generative AI im Bereich erste Hilfe” sowie “Generative AI als virtueller Patient” (WiSe 2024/2025)
- "Modellierung von Security-Aspekten von Fallbeispielen aus dem EU-Projekt FogProtect mithilfe ausgewählter Erweiterungen der BPMN" (SoSe 2021)
- "Privacy Metriken im Rahmen von FogProtectAnwendungsfällen" (WiSe 2021/2022)
- Abschlussarbeiten (siehe unten)
Frühere Lehrveranstaltungen:
- Organisation des Bachelor-Seminars am Lehrstuhl SSE (SoSe 2023 - SoSe 2024)
- Übungsleitung im Modul Requirements Engineering (WiSe 2023/2024)
Begleitete Abschlussarbeiten:
- Understanding Generative AI Usage: Daily Prompting Patterns and User Strategies (Bachelorarbeit Wirtschaftsinformatik, 2025)
- Potenziale von XAI-Methoden in der medizinischen Diagnostik: Identifikation von Faktoren, die Akzeptanz und Vertrauen bei medizinischen Fachkräften beeinflussen (Bachelorarbeit Wirtschaftsinformatik, 2025)
- Die Auswirkungen von Homeoffice auf die Cyberkriminalität (Bachelorarbeit Wirtschaftsinformatik, 2025)
- Refaktorisierung der Explainable-AI-Technik XRL-DINE zur flexiblen Anpassung an unterschiedliche State- und Action-Spaces sowie Reward-Channels (Bachelorarbeit Informatik, 2024)
- Anwendung der Explainable-AI-Technik XRL-DINE auf den adaptiven Videoencoder SAVE (Bachelorarbeit Informatik, 2023)
- Erweiterung des RADAR-Ansatzes für die Adaption von Fog-Computing-Systemen um eine Erklärbarkeits-Komponente (Bachelorarbeit Informatik, 2023)
- Automatische Erkennung von DSGVO-Datenschutzverletzungen in Laufzeitmodellen von Fog-Computing Systemen (Bachelorarbeit Informatik, 2022)
- Erweiterung von UMLsec zur Modellierung von Datenschutzanforderungen und -gefährdungen im Fog-Computing (Bachelorarbeit Informatik, 2021)
Mitgliedschaften:
- Bundeskader DLRG (seit 2018)